A Review of Statistical Reporting in Dietetics Research (2010–2019): How is a Canadian Journal Doing?
Abstract
Résumé
INTRODUCTION
METHODS
Checklist
Pilot testing
Data abstraction and analysis
RESULTS
Reporting of objectives and study design
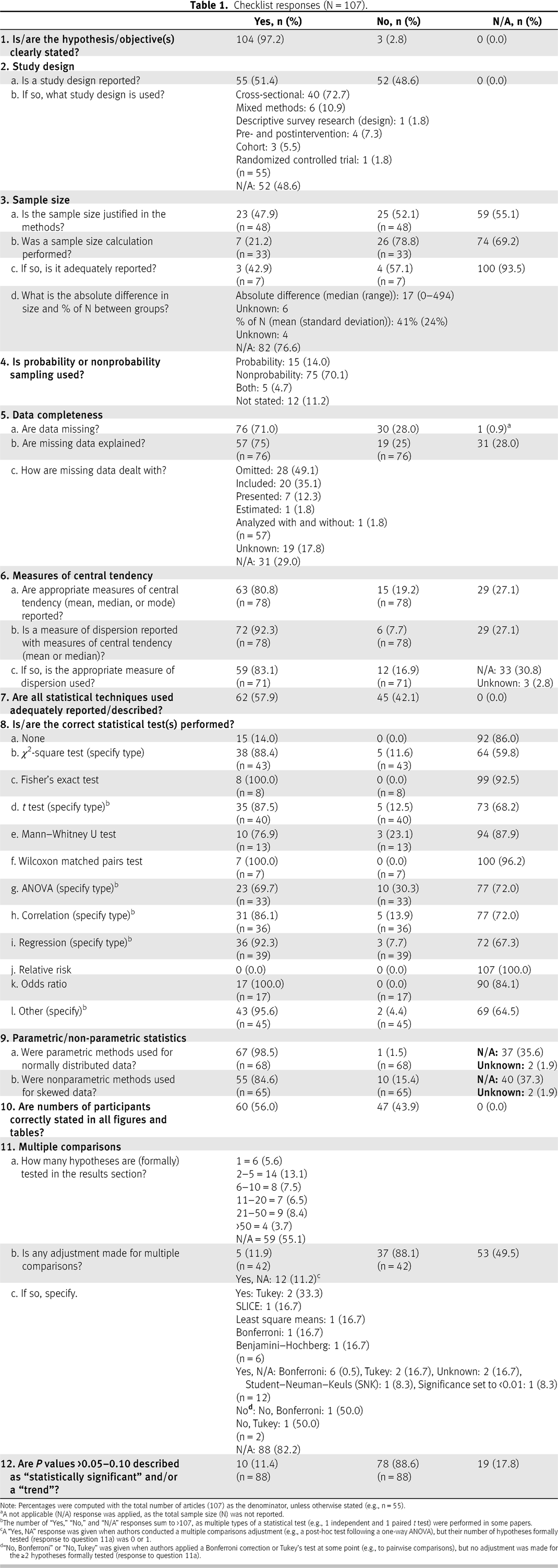
Note: Percentages were computed with the total number of articles (107) as the denominator, unless otherwise stated (e.g., n = 55).
Sample size
Missing data
Measures of central tendency and dispersion
Statistical reporting
Statistical methods
Sample size reporting on tables and figures
Multiple hypothesis testing
P value reporting
Changes over time
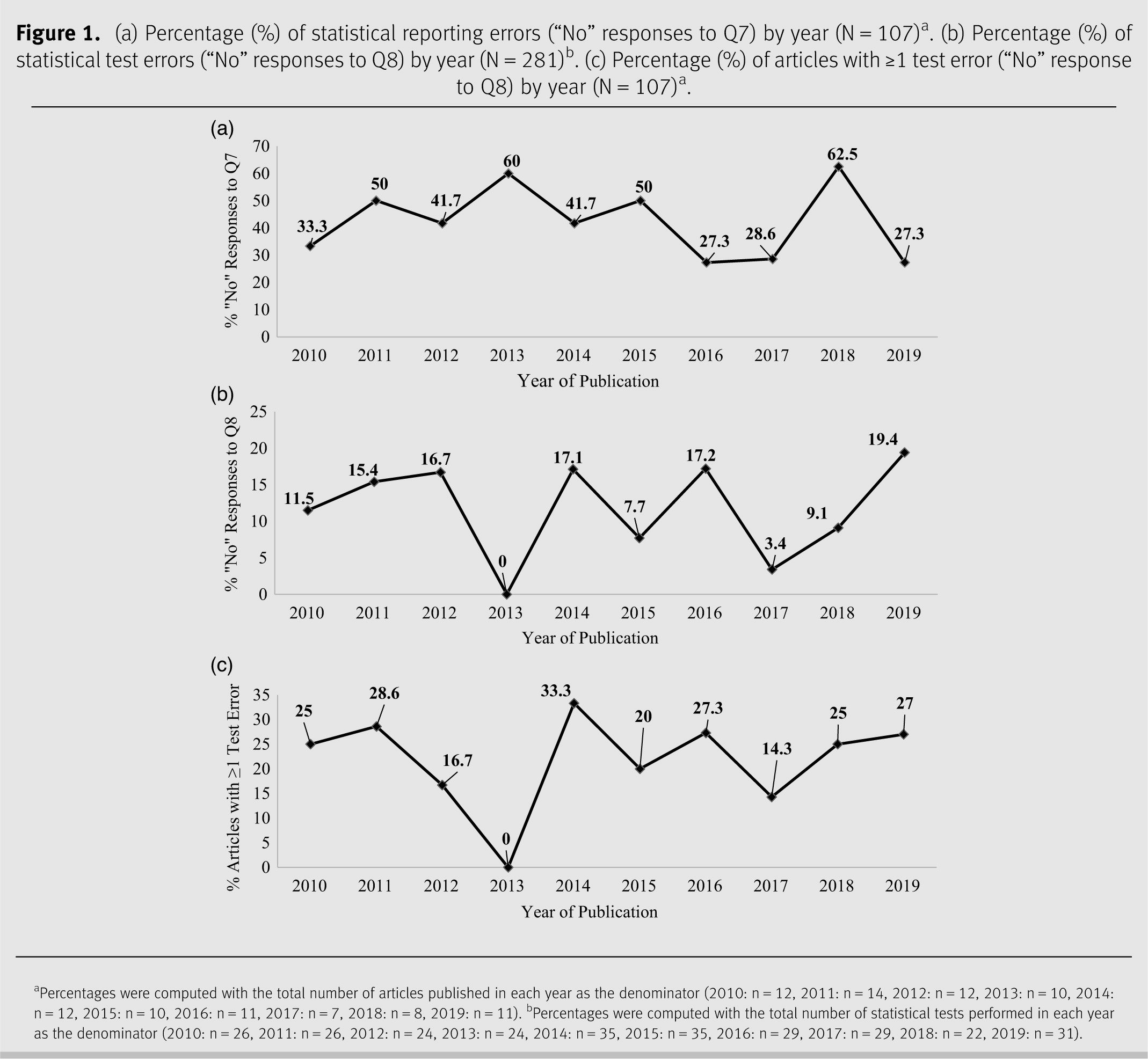
DISCUSSION
Statistical reporting
Statistical methods
Study strengths and limitations
RELEVANCE TO PRACTICE
Footnote
References
Supplementary Material
- Download
- 33.68 KB
Information & Authors
Information
Published In
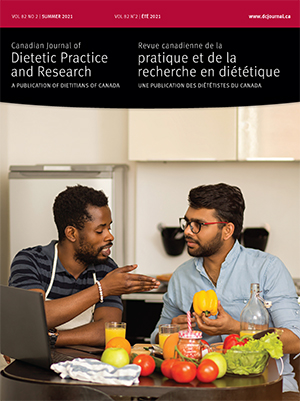
History
Copyright
Authors
Metrics & Citations
Metrics
Other Metrics
Citations
Cite As
Export Citations
If you have the appropriate software installed, you can download article citation data to the citation manager of your choice. Simply select your manager software from the list below and click Download.
Cited by
View Options
View options
Login options
Check if you access through your login credentials or your institution to get full access on this article.
Subscribe
Click on the button below to subscribe to Canadian Journal of Dietetic Practice and Research